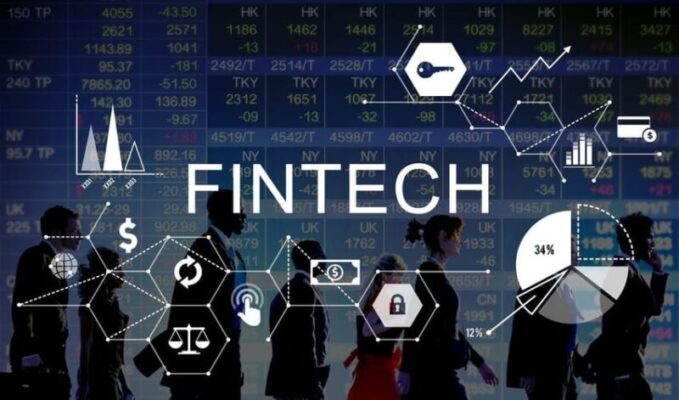
Applications of AI & Related Technologies in FinTech: 5 Use Cases
Last updated on April 16th, 2024 at 11:49 am
Banking and financial institutions have embraced technology as early as 1918 when the US Federal Reserve Bank developed the first electronic fund transfer system. Of course, later came the cash machine, the Diners Club credit card, the first ATM in London, and so on. But, a key point to note here is – the disruption all along was taking place within financial institutions or within the banking sector.
The real turnaround in the financial industry came when technologically advanced companies started emerging in retail financial services, in the consumer internet space. We’re talking mobile wallets, equity crowdfunding platforms, online lending platforms, and the like, which is now pushing legacy BFSI companies to innovate all over again.
What started as simple enhancements to banking services has now turned into a technology wave. Today, the most popular sectors in fintech are peer-to-peer lending, remittance, online payments, mobile wallets, and fraud, and security analysis. In 2019 alone, (according to CBInsights) fintech startups attracted investments close to the US $137.5 billion, with VC deals (early-stage) amounting to US $16.7 billion across 553 deals. The rest went into late-stage deals in the fintech space.
While technology has been at the core of fintech, the emergence of newer technologies like AI, machine learning, and big data analytics have propelled companies in this space to become more customer-centric. Today, the key goals for them is to deliver better customer experience, automate processes and optimize costs, enhance security, and build real-time data integration.
The Role of AI and Machine Learning in Fintech
AI and machine learning are co-dependent, meaning AI cannot operate without data, and the more data that is available, the better that machine learning can understand and churn out relevant information to deliver personalized experiences. That’s what fintech companies are looking to capitalize on now. They have vast amounts of customer data sitting in their transaction history, conversation history, account information, and more. Using this combination of AI and ML, there are several areas where innovation can occur; firstly, using past data and by analyzing human behavioral patterns, AI and ML can help companies detect and assess frauds. Secondly, AI-based chatbots can reduce customer dependence on human interactions, ensuring speedy resolution, thus improving operational efficiency and increasing employee productivity. Thirdly, companies can build highly personalized campaigns to attract new customers and build loyalty among existing customers. Aside from these, they can also help companies offering credit services assess the creditworthiness of the customer.
Going forward, AI and ML are capable of taking the customer experience to the next level. Let’s look at five use cases where AI and ML are disrupting the fintech industry.
Use Case #1: AI for Credit Risk Analysis
There are several internal and external factors that are driving financial institutions to move away from the traditional methods of assessing risk, to adopting faster, more efficient means to deal with credit risk. Externally, there are increasing regulations and the emergence of newer fintech companies threatening to disrupt this space. Internally, there’s a mercurial demand from customers to move away from the traditional customer risk management process by taking advantage of big data analytics. There’s also an increased focus on reducing risk from non-performing assets.
AI and ML can play a pivotal role in credit risk analysis and management. On one hand, it can identify high-risk or low-risk customers, customers who are likely to default on loans, and the like. On the other hand, instead of following a rigid process, ML can provide more accurate insights as more and more data is fed into the system. For example, in the pre-qualification stage, AI and ML can qualify customers, even those with limited data, based on occupation, income, and address. Then, it can analyze the documents sent by the customer, and alert teams of any discrepancies. Across all stages, NLP can engage in contextual conversations with customers and assist them with relevant information to initiate the lending process. In fact, aside from analyzing and determining customer credibility based on structured data, AI and ML can also access unstructured data such as geographical information, social media data, past loan payment trends, and such, to help companies reduce the risk of default, and award appropriate credit scores for borrowers.
Use Case #2: Chatbots for Customer Support
With the adoption of AI and NLP, fintech firms are taking customer experience one step further by having intelligent chatbots engage with customers across a spectrum of services. For example, chatbots can access historical data and respond to customer queries within minutes, they can handle multiple queries at a time, and they are available 24/7. Moreover, with advancements in NLP and machine learning, chatbots are able to access more customer data and understand human communication trends, to respond to complex queries with more accuracy.
When a layer of product recommendation is added, the bots can suggest relevant products or services offered by the business, based on the customer query. A recent study suggests that with chatbots, financial institutions can potentially save 826 million working hours by 2023, and save the US $7.3 billion in operational costs.
Use Case #3: AI for Personal Finance & Robo Advisory
In the peer-to-peer payments space, chatbots can assist customers to make transfers, pay bills, check account balance, and more, seamlessly. They can also help customers manage money better, just as relationship managers do, by reminding them of budgets they have set for themselves, by showing them their spending history and helping them make more informed decisions.
In fact, they can also act as personal finance advisors by accessing the customer’s transaction history and spending patterns, and suggesting money saving strategies, or act as Robo advisors, recommending areas where customers can make micro-investments to earn better returns.
Use case #4: Automation of KYC
KYC regulations play a vital role in protecting banks and customers from being exposed to financial frauds and corruption. It is a key process to ensure compliance with AML regulations. That being said, KYC processes are often complex, time-consuming, and vary depending on geography.
A report reveals that banks spend up to the US $500 million on KYC and customer due diligence every year, onboardings take up to three months, and the elaborate processes involved often frustrate customers and push them to move to other banks.
Thankfully, with advancements in AI, there’s potential for KYC to become quicker and intuitive. On one hand, given the vast access to customer data across departments, systems, and sources, AI can help set a credit limit for customers, and make the account opening process more seamless, thus eliminating the human error involved in analyzing tons of paperwork and information. Moreover, with AI continuously monitoring customer transactions and account behavior, it can ensure that compliance losses are reduced, and regulatory norms are met. Of course, with significant automation of KYC processes comes reduced dependency on manual processes, which in turn reduces annual costs of compliance for fintech companies.
Use Case #5: Automation of Claims Processing
Typically, if a customer buys an insurance (health, motor, or car insurance), he/she subscribes for the policy, and in the event of damage, seeks a claim. Traditionally, the claims process is time consuming, complicated, involves tons of paperwork, and manual effort by the underwriter and the customer.
AI has the ability to reduce the manual effort involved in claims processing, thus reducing human error, saving operational costs, saving time, and making the journey more pleasant for the customer and the underwriter. For example, it can take the customer through the entire claim process with step by step instructions. It can acquire the necessary documents for the claim and analyze them to ensure that they are accurate and compliant. It can also detect frauds if any. Then, it can suggest various payout options for the claim, and allow human intervention, once all information has been acquired and verified. A report by Accenture shows that insurance can achieve 30% more accuracy when they automate the classification process. In turn, it can also improve employee productivity by 80%.
Anvika Sharma is a Senior Software Engineer in Mobinius Technologies – a leading Digital Transformation Company. She is passionate about computers and technology and loves to write on various topics and express her thoughts in the form of write-ups.