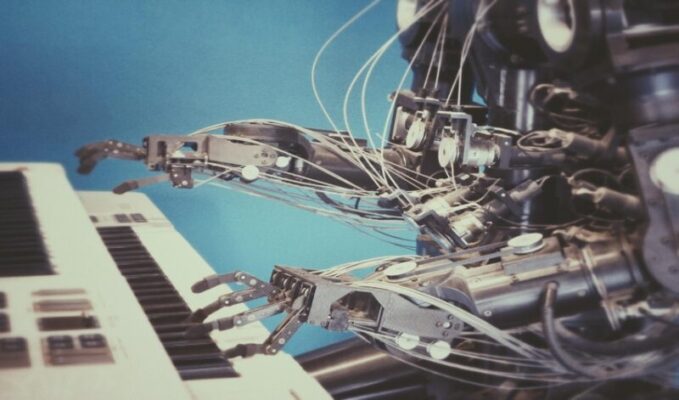
Misconceptions about artificial intelligence
Every day we hear that artificial intelligence will solve all our problems – from self-managed cars to cancer treatment. At the same time, some scientists and industry captains, such as Elon Musk, founder of Tesla, believe that artificial intelligence poses an existential threat to humanity. Where is the truth and what is hidden under this term? The hype around it is only beneficial for attracting investments. The same Elon Musk is a marketing genius, but even he does not know everything about AI.
History of development of the concept of AI
The term “artificial intelligence” was born in the 50s of XX century, and already then there was a debate about what it means. The first systems for text editing were considered “smart”. That’s when the joke was born that artificial intelligence is what people can do, but computers are not yet. That is, originally artificial intelligence was considered as automation of human mental activity.
In the 80s of the last century, the so-called expert systems became very popular. They had a great impact on the automation of business processes, which are governed by precise rules. Once, the application of business rules was followed by the army of managers. Then, these rules became part of the code of management programs. Under the influence of expert systems, they were separated from the code and collected in tables. In modern management systems, it is possible to change the rules without reprogramming the system itself.
The hype around the AI has peaked and is now in decline – its capabilities have been overrated. Such peaks are called the “summer of AI“, and drops – when the technology begins to doubt – the “winter of AI“. This is due not only to the fact that futurologists’ prophecies have not yet come true. And not fear of AI: in addition to the paranoia caused by a possible “revolt of the machines”, more realistic overheating of the planet at the expense of the energy required by the machines for data processing and training is also frightening.
With these examples, we can see that mental activity automation systems do not learn anything themselves. All their knowledge, such as expert rules, must be developed and entered manually. Recently, the focus has shifted to so-called machine learning systems. The purpose of these systems is to replace manual rule development with automatic learning by examples. Until the end of the 1990s, machine translation systems worked on rules developed by dozens of linguists. The success of these systems left much to be desired. With the spread of the Internet it was possible to collect a large number of parallel texts in two languages. So were proposed statistical models of translation. The parameters of these models were automatically optimized on the basis of parallel texts without the use of linguistic rules. The same type of work took place in the direction of speech recognition. This approach gave a big leap forward in the quality of translation, once the number of examples for training reached tens of millions.
Modern statistical machine learning systems based on deep neural networks have achieved impressive results in machine translation, speech recognition and photo analysis. This leads optimists to believe that there is a cure for cancer and smart robots to talk to on any subject. Pessimists talk about mass unemployment and even about uncontrolled robots capturing the world. Both of them run far ahead, in the field of science fiction.
Myth 1: AI is able to solve any problem
All modern artificial intelligence systems are highly specialized. Over the years, lots of systems have been created that automate certain types of human mental activity, for example, playing chess or recognizing handwritten words. But even the most perfect chess program will not be able to answer the question of where the current world champion Magnus Carlsen was born or create a picture or a symphony as in the movie “I am a Robot”, fortunately there are cool services like TakeTones where creative personalities create that very symphony. She can only make chess moves – nothing else. We do not yet know how to create systems of general, rather than highly specialized, intelligence.
IBM tried to make a marketing campaign out of this, based on the idea that if a computer can win at chess, it can do anything, like treat cancer. In fact, this is not the case. At the present stage of development, various methods of artificial intelligence can solve individual problems, and quite successfully. But the theory of general intelligence still doesn’t exist.
Myth 2: AI all can do it themselves
Statistical machine learning systems require a huge amount of marked data – parallel texts or pictures with selected subjects. There are not many areas in which such data exist. Insufficient examples of training lead to a large number of errors.
Our extensive knowledge and logic allow us to learn from very few examples. Psychological experiments show that one picture of an antelope is bent enough for a person to learn to recognize it. And this is for a person who has never seen an antelope bent before. For the best neural networks, you need thousands of photos. Most likely, people can do it faster and better, because they have rich ideas about animals with which they can compare something new. As Pushkin used to say, “science reduces the experience of fast life”.
Conclusion: why is AI unequal to human intelligence?
What is missing for the construction of general intellect? We do not have a clear answer to this question. By analogy with human intellect, it is worth paying attention to several necessary components. It is often said that lack of artificial intelligence has common sense. But what is common sense? It is our knowledge and the logic of its application. A two-year-old child does not need to touch a hot stove ten times to be afraid of it. He already has a model of hot objects and an understanding of what happens in contact with them. It is enough to burn once to not want to touch such objects anymore.
How does our knowledge get into our heads? Very little of what we know has come to us from our own experience. From early childhood we learn thanks to people around us. Our knowledge is collective, our intellect is also collective. We help and prompt each other all the time. This concept does not exist in modern machine learning systems. We can’t tell a neural network anything, and it can’t teach us anything. If one robot learns to recognize a sheep and the other a cow, they can’t help each other.
Until we solve these problems, we do not need to be afraid of ubiquitous robots. Rather, we should be wary of myths about artificial intelligence and blindly follow the instructions of far from perfect machines. However, the same can be said about people.
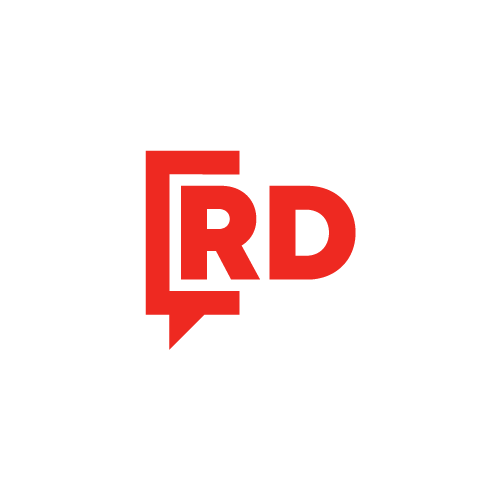
Read Dive is a leading technology blog focusing on different domains like Blockchain, AI, Chatbot, Fintech, Health Tech, Software Development and Testing. For guest blogging, please feel free to contact at readdive@gmail.com.